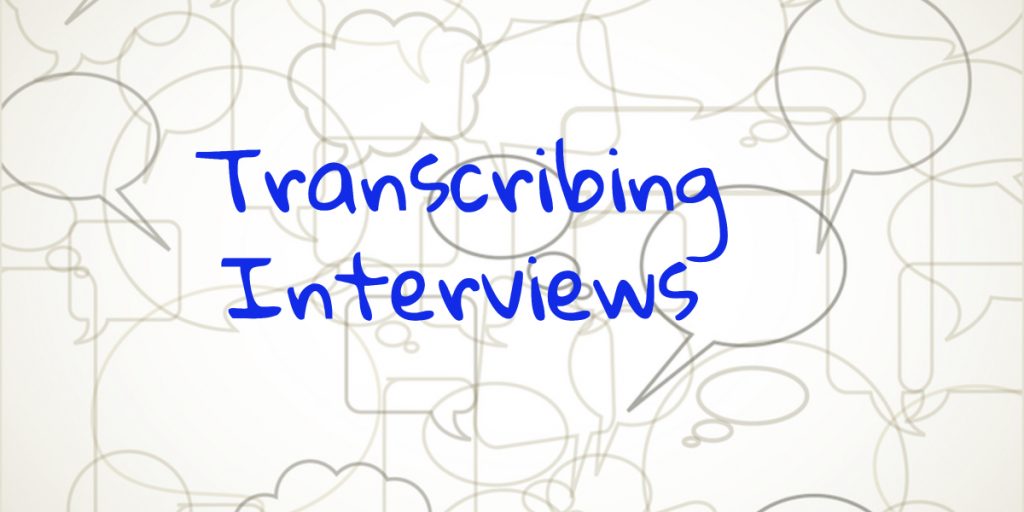
The Magic, and Limits, of AI-Based Speech Recognition
If you interview people, on a podcast or for research, you have probably faced the problem of interview transcription: turning that audio format into a written form.
Do you take notes? Transcribe the interview yourself? (That’s only advisably if you’re an excellent typist.) Hire a transcription service?
There’s another option: using speech recognition technology to transcribe the audio for you.
If the term “speech recognition” makes you remember screaming futilely at an automated voice mail system (“SPEAK TO AN AGENT!”), it’s time to think again.
Technology has come a long way with understanding human speech.
In the course of researching my next book, I’ve been interviewing a bunch of authors. I have hours and hours of interviews and wanted to make clean transcripts of them as fodder for my book research. I also wanted to share excerpts of some of them publicly, because there’s much more in the interviews than can land in the book. And along with the recording, I want to share the transcript, as not everyone has time to listen to 20-minute interviews.
I quickly discovered that interview transcription is hard! It takes time. I started by doing it myself, listening to the audio track while typing furiously (and hitting pause all the time to catch up.) Even though I am a fast typist, a 25-minute interview might take well over an hour to transcribe with any accuracy.
AI-based speech recognition to the rescue!
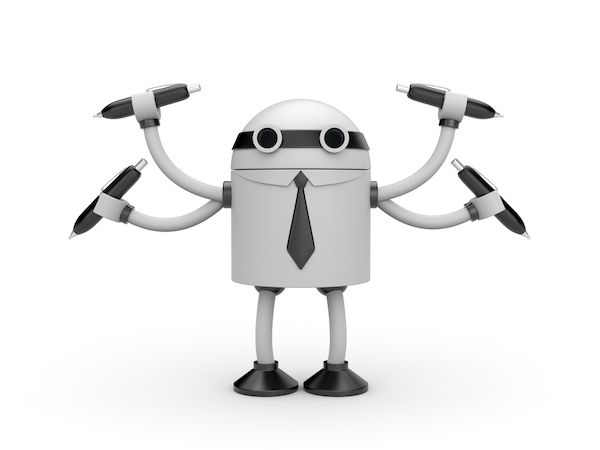
Experimenting with Otter’s AI-based solution
Productivity coach Judy Dang recommended that I experiment with Otter.AI, an online platform that uses artificial intelligence technology to create text representations of conversations.
Otter can transcribe conversations live, as they happen, or work with audio files that you upload to the platform. It also integrates with Zoom to record those endless Zoom meetings.
Otter offers both free and premium, paid services. I experimented with the premium service.
The software does a remarkably good job of creating an interview transcription in near real time, as a conversation occurs. It makes excellent guesses about words, even assigning them to different voices. (Although, it often missed the handoffs between me and my interview subjects, also women.)
As you might expect, it was easily confused by unexpected or unusual words.
- When transcribing a webinar on finding your book’s niche, it changed many of the niches to nice.
- I used it on an interview I did with April Rinne about her upcoming book Flux Mindset. April, Otter suggests that you write a book on a flex mindset instead.
- It was befuddled by the way that Michele Wucker kept dropping the phrase “gray rhino” into conversations about books, risk, and finance. (Michele has a book of that name.)
I almost felt bad for the AI bots trying to sort through our conversations.
But those issues were easy to fix. The larger challenge with using speech recognition technology has nothing to do with the technology, and everything to do with human speech.
Speech and conversation are different
Because these interviews took place over video, they felt like a personal conversation. (That was intentional on my part.)
Human conversation is messy.
There’s a huge difference between issuing short commands to Alexa or dictating text messages to Siri and a having a lively exchange with another person.
None of us speak in perfect sentences. We interrupt ourselves, wander off …. And we repeat ourselves. We interrupt each other and laugh at each other’s jokes.
Many small exchanges grease the wheels of conversation—little check-ins (right?) and confirmations. We deploy verbal space-fillers while our brains process how best to phrase a complex idea.
An accurate, word-for-word transcription is filled with clutter.
Although I don’t edit that clutter out of the audio files, I do want to clean up the transcript, to serve many interests:
- Interview subjects: To honor and respect the time they spend with me, I can at least clean out the conversational clutter and let their ideas rise to the top.
- Readers: The reader shouldn’t have to wade through small byways, repetitions, and interruptions.
- Myself: I want to sound pithy and wise as well. Believe me, after listening to these transcripts, I am well aware of my conversational tics!
It takes me many revision passes to clean up the automated transcripts to meet my own standards.
Human vs. automated interview transcription
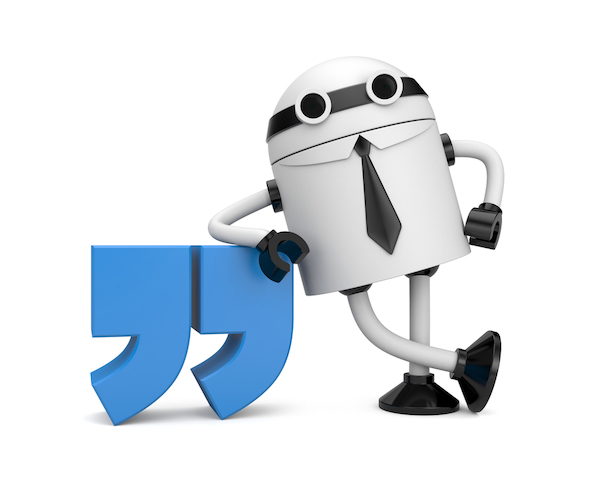
After several interviews, I had a surprising realization: the software didn’t save me time, it merely changed how I spent my time on these transcriptions.
When I transcribed manually, listening and typing and hitting pause, I could clean up the text as I went. I’d listen to a sentence, then type it out (and perhaps double-check it.) I made a few mistakes, but they were the type easily found with spell checking. I wouldn’t even type in the false starts, the repetitions, or speech mannerisms.
In contrast, Otter gave me complete scripts requiring extensive editing. That felt harder to do. In editing out the excess verbiage, I introduced new errors, which were then difficult to see. Each of these efforts required multiple revision passes.
Typing the transcript myself took about the same amount of time as fixing the automated transcript overall. But working on the text directly yielded other benefits:
- In transcribing, I re-live and internalize the conversations. I identify the main themes and organize the ideas for my book research.
- Typing the transcript gives me the chance to discover and highlight quotes that I want to use, either in the interview post or as potential fodder for the book.
When I’m done, I have a better understanding of the content. For me, it’s worth the effort of typing.
A blended approach to interview transcription
If you’re wrestling with this issue, experiment and see what works for you.
- For interviews in specialized fields, find a transcriber who is familiar with the vocabulary used, or use the premium version of Otter and “train” it to understand your terminology, proper names (or book titles)!
- If you want to publish the transcription with speakers appearing in a positive light, use a human transcriber (or do it yourself). Let the transcriber know that you don’t the repeated words and mistakes. Even if you end up editing, you’ll be starting with something cleaner.
- The automated transcription works well for files you’re using solely for research, as you can ignore the clutter. Highlight the bits that interest you most, and disregard the rest.
My personal approach: I’m manually listening to and writing up the interviews I plan to publish. When I want to turn an audio file into notes for internal research, the software is terrific!
Have you experimented with automated interview transcription? Or speech recognition for drafting? I’d love to hear about your experiences.
You might also like:
Check out the interviews I’ve been doing here: Interviews with Inspiring Authors
If you’re interested in speech patterns, here are a couple posts that you might like: